Robotic neurorehabilitation for the upper limb – new insights

Authors: Mihaela Molnar, Oana Vanta
Keywords: robotic devices, neurorehabilitation, upper limb motor rehabilitation
Introduction | Robotic neurorehabilitation for the upper limb
Chronic and incapacitating neurological conditions significantly burden families and society [1]. Brain injuries and other neurological pathologies can negatively impact a patient’s life quality by causing motor or sensory loss or dysfunction [2]. One of the leading causes of mortality and disability around the globe is stroke [3]. After a stroke, patients frequently have sensorimotor impairments, the upper limb is affected in more than two-thirds of affected individuals, and half of them experience a persisting loss of arm function [2].
Spasticity in the upper extremities affects 17% to 40% of stroke survivors, making it harder for them to perform activities of daily living (ADL). Upper-limb therapy is essential in the first six months following the stroke because, after that time, stroke survivors’ motor and ADL recovery diminishes. After six months following a stroke, 33% to 66% of individuals do not regain functional use of their upper extremities [3].
The importance of robotic neurorehabilitation
Innovative robotic devices have been created over the past few decades to assist physicians in neurorehabilitation [2]. Traditional post-stroke rehabilitation methods include:
- “hands-on” therapy (manual therapy techniques)
- constraint-induced movement therapy
- repetitive task training
- mirror therapy.
They frequently call for patients to manually perform a partial or full-assisted movement in arm/hand joints while being observed by therapists.
Robot-assisted treatment
The cost-effectiveness of traditional therapeutics has been constrained by how labor- and time-intensive they are. Robotic devices are used in robot-assisted treatment (RT), a revolutionary post-stroke rehabilitation strategy, to give patients motor or task-oriented training. Stroke survivors can perform independent training with less supervision from therapists, receive timely feedback on their performance from robotic devices, and achieve better adherence to treatment with the introduction of games or interactive upper-limb tasks, in addition to providing repetitive and high-intensity training in a cost-effective manner [3].
Robotic neurorehabilitation is appealing due to its ease of deployment potential, adaptability to various motor impairments, and excellent measurement reliability [4]. One of the major objectives of rehabilitation for people with neurological diseases is to increase mobility. High dosage and intensity, sufficient practice, specific objectives, motivation, and specialized expertise are all crucial for improving outcomes in neurorehabilitation [1].
Robotic-assisted gait training (RAGT), which may deliver a stronger treatment dosage than standard rehabilitation, is anticipated to increase mobility more successfully than conventional therapy [1]. In this application sector, “robotic technology” refers to any mechatronic device with a certain level of intelligence that may physically influence the patient’s behavior to optimize and improve his or her sensorimotor rehabilitation [2].
These robots have two main functions:
- Evaluate human sensorimotor function
- Retrain the human brain to enhance the quality of life [2].
Therefore, based on the low-level control method and every patient’s remaining abilities, each robotic device provides a pre-defined training mode. Both passive training (robot-driven, position control technique), where the robot imposes the trajectories, and active training (patient-driven), where the robot modifies its trajectory in response to the subject’s will to move, are often implemented by rehabilitation devices.
Riccardo Iandolo et al. indicate the fact that the supportive training modality, however, is the most pertinent of all the other training methods. In the active assistive training mode, the assistive controllers, which mimic the traditional physical and occupational therapy approach, aid participants in moving their impaired limbs into the proper positions when grabbing, reaching, or walking [2].
Particularly, the assistance-as-needed approach is one of the assistive techniques frequently used since it lowers the chance that the patient would only rely on the robot to complete the rehabilitation job.
On the other hand, Riccardo Iandolo et al. point to the fact that over-assistance may, in fact, reduce engagement and, hence, the potential for inducing neuroplastic alterations [2]. The “Slacking” effect, for example, develops when a patient undergoes repeated passive limb mobilization, which is defined as a decline in voluntary movement control. Challenge-based controls are used to make tasks more challenging or stimulating in addition to the assistance-as-needed strategy to stop the ‘slacking’ effect [2].
The most effective use of currently available technologies or gadgets would be a major study emphasis, even if numerous studies are concentrating on developing new ones. This might be accomplished by developing effective training techniques and implementing realistic control and assessment methods [2].
In order to optimize the potential of neurorehabilitation, robotic treatment must be combined with other disciplines, including computational neuroscience, motor learning and control, and bio-signal processing, among others [2].
ROBOTIC DEVICES USED IN UPPER LIMB MOTOR NEUROREHABILITATION
A therapy robot can be referred to as a multipurpose manipulator that can be reprogrammed and used for various rehabilitation tasks. Therefore, it would be feasible to put up a robotic system that moves the arm and hand to conduct this function, similar to locomotion robots used for gait therapy. In contrast to the lower extremity, there is no established typical motion pattern for the upper extremity, where a particular gait pattern may be identified. It requires a distinct approach due to the intricacy of the arms and hands and the wide range of motion patterns that are accessible [5].
Based on the different forms of physical human-robot interaction, there are two primary categories of robotic devices for neurorehabilitation:
- End-effector devices;
- Exoskeletons [2];
End-effector-based systems are robotic devices equipped with an exclusive interface that mechanically restrains the distal portion of the human limb, which is directly controlled; the rest of the kinematic chain is free, and the human limb has to adjust to external disturbances or movements performed by the end-effector robot [2].
Exoskeletons faithfully mimic the kinematics of the human limb and support its motion by manipulating the position and orientation of each joint. Additionally, the number of actuated joints and the range of motion (ROM) are properly selected to maximize control, resulting in close monitoring of the patient’s motion but at the cost of a higher complexity for the control of degrees of freedom (DOFs) [2].
In the past years, numerous robotic systems and protocols have been created based on task-oriented repetitive motions for the improvement of:
- ROM;
- Muscular strength;
- Movement coordination;
- Motor learning [2].
Based on the nature and degree of motor dysfunction and associated disability, one type of device may be more effective than the other; for example, exoskeletons may be more suitable to deliver forces to each joint if the patient has very low remaining sensorimotor functionality [2].
The following table exemplifies some neurorehabilitation devices (Table 1) [2,6].
End-effector robotic devices | |
MIT Manus | designed for the shoulder and elbow joints |
ARM (Assisted Rehabilitation and Measurement) Guide | a counterbalanced robot that assists the reaching motion mechanically without loading the arm |
GENTLE/s (Robotic assistance in neuro and motor rehabilitation) | |
Italia NeReBot (Neurorehabilitation Robot) | mainly utilized to quantify aberrant joint torque coupling in chronic stroke |
ACT (Arm Coordination Training Robot) | mainly utilized to quantify aberrant joint torque coupling in chronic stroke |
Mirror Image Motion Enabler | implement bimanual training protocols |
Bi-Manu-Track | implement bimanual training protocols |
Exoskeletons devices | |
SUEFUL ARMin III CADEN (Cable-Actuated Dextrous Exoskeleton for Neurorehabilitation) RUPERT (Robotic Upper Extremity Repetitive Trainer) | Provide shoulder and elbow joint motion |
Manovo Power ARMEO Power IntelliArm exoskeleton | Can implement movements like hand opening and closing, grasp movements, fingers passive stretching |
Table 1. Examples of exoskeletons and end-effector devices ( available from [2,6])
In contrast to end-effector devices, upper limb exoskeletons for rehabilitation have recently been created due to the following:
- the intricate interaction between the mechanical structure of exoskeletons and the various joints in the human body,
- the intricate control schemes that must be adopted to deal with transparency and back-drivability,
- the requirement to promote patient sensorimotor recovery without passively moving the patient’s joints by using assistive training modalities capable of responding to any pathological movement [2].
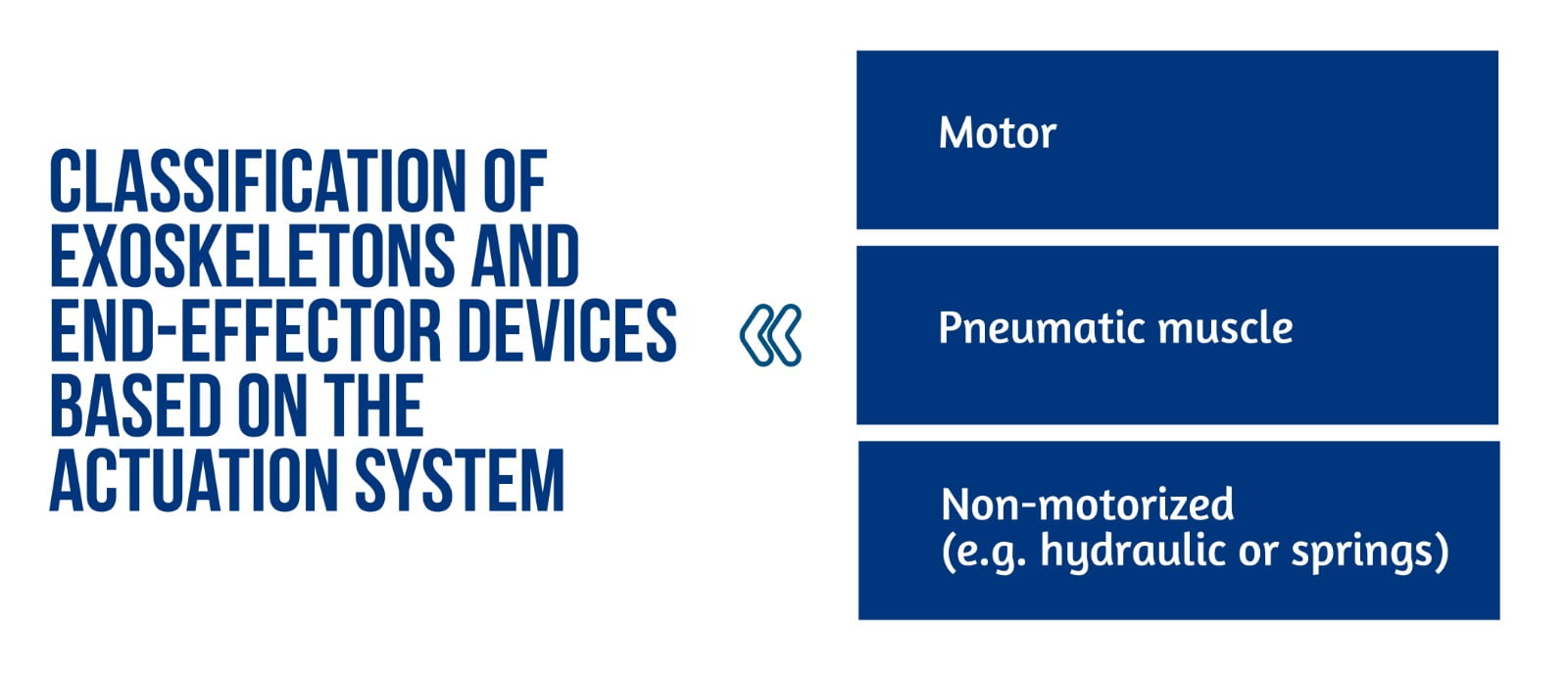
Several innovative control schemes for exoskeletons have recently been designed to improve inter-joint coordination [2].
End-effector and exoskeleton devices (Figure 1) implemented a closed-loop feedback control with feedforward components. This approach allows for correcting patients’ performance faults and compensation for the device mechanics’ weight, inertia, and friction. Furthermore, the robot model might generate the feedforward components or learn iteratively. This control method is typically used in exoskeletons, where position data is used to close the loop. The interaction control framework is also used to implement assistive methods. Most end-effector devices, in particular, use impedance control techniques, whereas exoskeletons use admittance control schemes [2].
The exoskeleton can function in three modes: passive (robot-driven), active (patient-driven), and challenge (robot resists applied force). A robot may also oppose the patient’s movement to make things more difficult for the patient [6]. Sliding mode controllers or controllers activated by the patient’s intention detection estimated by electrophysiological measures (e.g. surface electromyography – sEMG) and electroencephalography – EEG) have been developed in recent years for upper limb exoskeletons.
All of the approaches described above are based on comparing an error signal to a reference trajectory which may be easily estimated or created with end-effector devices; however, exoskeletons present several challenges. As previously stated, exoskeletons can restore patient inter-joint coordination by appropriately adjusting the various robot joint trajectories. Nevertheless, the relationship between recovery and exoskeleton trajectories remains unknown [2].
Recently proposed approaches include:
- Reproducing previously recorded trajectories made by healthy participants;
- Using previously recorded pathological involuntary joint torques translated in the joint kinematics domain [2].
A brief introduction to wristbot
WristBot (see Figure 2) is a robotic end-effector device intended for the neurorehabilitation of individuals who suffer neurological or orthopedic diseases allowing movements of flexion/extension in radial or ulnar deviation and pronation or supination. It has four brushless motors that allow it to guide and aid wrist motions in the three planes stated above. These motors are chosen to give precise haptic representation while compensating for the device’s weight and inertia, allowing free and smooth motions [2].
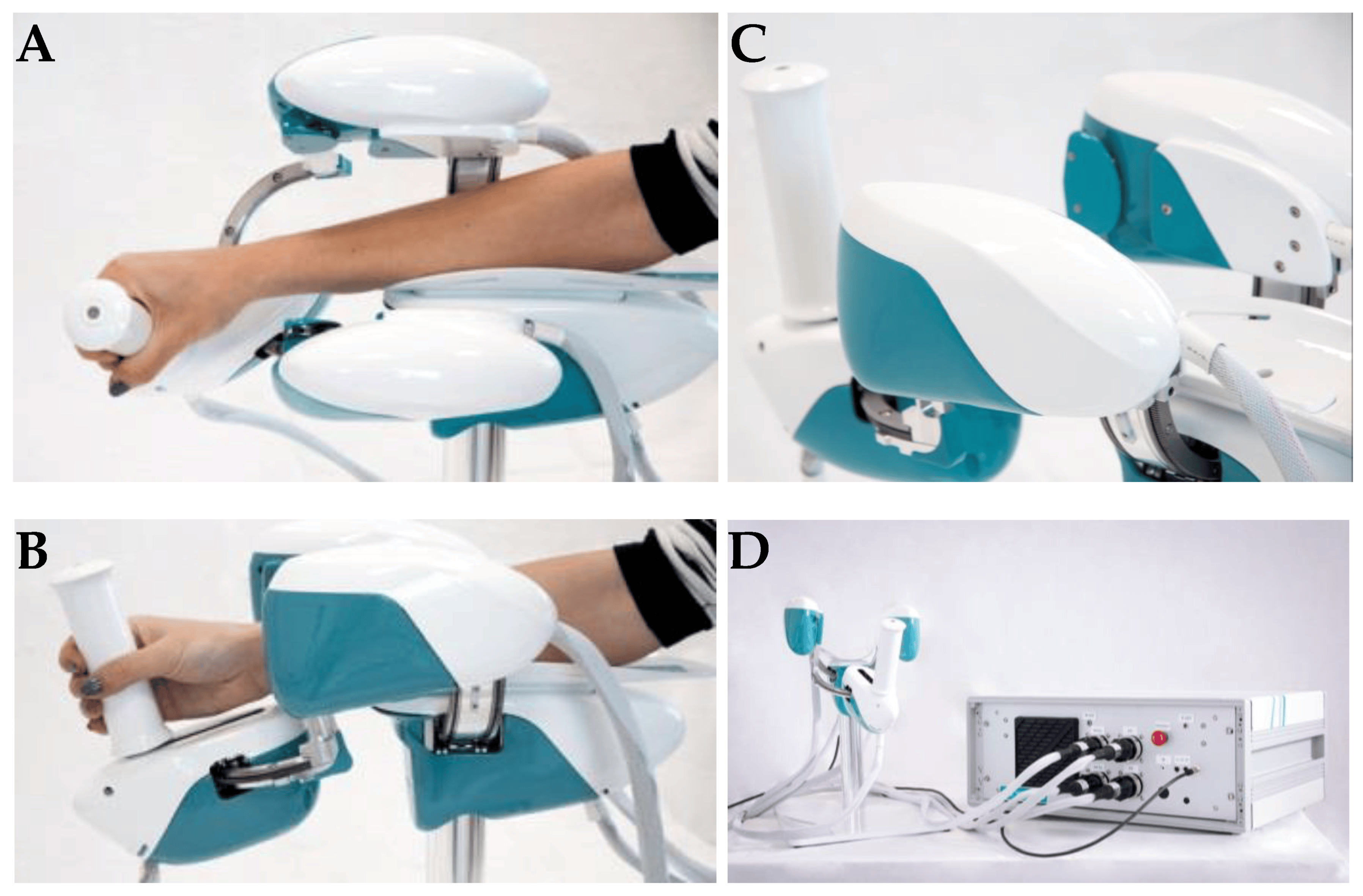
The key benefit of the WristBot is its highly customized therapy, provided by its versatility and programmability. Additionally, the device’s quantitative functional evaluation is a helpful tool to aid physicians in selecting the best course of treatment [2].
Latest advances in neurorehabilitation involving robotic devices
The neurorehabilitation process greatly benefits from robotic training. In fact, the devices may be programmed to execute several training options depending on motor learning paradigms and/or brain control. Robots can also read out information on movement performance with accuracy and precision and offer highly repeatable, exact, and reproducible movements (as dictated by forces and torques). Riccardo Iandolo et al. highlights that a suitable rehabilitation training regimen must be carefully created to make the most use of rehabilitation robots for patient care. To do this, a tremendous amount of research has been undertaken in recent years to fully understand the many mechanisms by which people (re)acquire (or relearn) a motor skill [2].
Planning an effective robot-based treatment to promote sensorimotor rehabilitation requires a thorough understanding of how the brain governs movement and which applied processes are used to learn new abilities [2].
A key factor in facilitating motor learning is motor variability. Variability was once regarded as “noise” that the brain needed to eliminate when learning, but recent research has begun to recognize its significance in developing motor abilities [2].
The benefits of receiving visual feedback from seeing someone else do an action have been amply established to enhance motor learning. According to recent research, respondents learn better when they see a video of someone executing reaching actions in a complex setting before performing the activity on their own. Motor and sensory learning are tightly connected, in the same manner, that motor learning influences sensory networks and sensory learning alters motor regions [2].
BCIs (Brain Computer Interfaces) were first designed as non-invasive communication aids, but their intrusive counterpart, commonly referred to as brain-machine interfaces (BMIs), seek to restore some degree of motor function in patients who were entirely paralyzed or severely disabled. Recent studies demonstrate how BCIs and BMIs may be effectively used to improve the results of a neurorehabilitation intervention: these systems advocate for help that mirrors the user’s motor intention, leading to greater cortical modifications.
The findings open the door to innovative, tailored therapies where closed-loop decoding of brain activity is crucial for enhancing sensorimotor repair [2].
Multidisciplinary evaluation of recovery – assessment of sensorimotor performance
The current clinical approach for assessing movement disorders mainly comprises qualitative assessments made by human operators using clinical scales, as shown in Table 2 [2].
CLINICAL RATING SCALES | |
Quality of Upper Extremity Skills Test (QUEST) | Assesses movement patterns and hand function |
Modified Ashworth Scale (MAS) | Assesses the spasticity of the upper limb |
Fugl–Meyer Assessment (FMA) | Evaluates sensorimotor impairments |
Melbourne Assessment of Unilateral Upper Limb Function (MAUULF) | Evaluates the quality of the movements |
Box and Block Test (BBT) | Evaluates gross manual dexterity |
Table 2. Clinical rating scales
The following tests evaluated position sense acuity, detection of passive motion, and kinesthesia: Nottinghan Sensory Assessment, Rivermead Assessment of Somatosensory Performance, and Joint Position Matching test [2].
Sensorimotor performance can also be measured via brain and/or muscle activity recordings such as high-density electroencephalography (hdEEG) recordings, source imaging methods that have lately enabled the accurate reconstruction of resting-state networks in the brain, and assessing of electrophysiological sub-cortical activity [2].
Functional connectivity (FC) represents the relationship between separate brain areas and changes after proprioceptive training with a robotic device. Moreover, FC predicts the behavioral outcomes of rehabilitation protocols and motor function recovery in stroke patients and corresponds with the extent of clinical impairment in patients with early relapsing-remitting multiple sclerosis [2].
The promises of robotic devices in neurorehabilitation
Because motor impairment is the common denominator of all functional motor disorders, focusing rehabilitation efforts on the restoration of impairment rather than compensation could be more beneficial in the acute and subacute stages of recovery. High intensity (i.e., dose per unit time), high dosage, and realistic movement training in these three dimensions are important factors influencing the impact of rehabilitation [4].
Robotic neurorehabilitation equipment may increase the quality of the quantitative evaluation, which is also necessary to comprehend better and infer the rehabilitation therapy’s effect on sensorimotor function. Robotic measures have the potential to exceed human-administered clinical scales and are only limited by the robot sensors’ performance [2]. Robots provide a more exact evaluation of both initial impairment and impairment changes in response to therapy regarding movement kinematics and dynamics [4].
Conclusion
Future research should concentrate on creating novel mechatronic structures and improved control algorithms. Exoskeletons must cope with a high level of mechanical complexity: they must be portable, lightweight, efficient, and compliant while also properly supporting the patient, even in the presence of severe impairments. In order to control costs throughout the design of a device, it is essential to keep in mind the specific disability the device is being developed to choose just the most crucial developmental goals [2].
Through the advancement of robotic scales and the incorporation of measurements with biosignals, sensorimotor performance, and recovery may be comprehensively evaluated. Researchers propose that new methods based on multimodal evaluation methodologies should be used to leverage unique and/or composite indications. This may result from understanding how to provide the greatest tools to improve motor recovery and neuroplasticity [2].
The study of Riccardo Iandolo et al. [2] highlights the importance of patient evaluation regarding rehabilitation intervention to increase its effectiveness. When building a tool to help a certain disorder, it is critical to consider the end perspective, as the user’s collaborative effort would almost certainly result in a proper treatment [2].
Robots provide movement controllability and measurement reliability, making them excellent tools for neurologists and therapists in addressing neurorehabilitation issues [4]. According to studies, robot-assisted treatment is less expensive than standard intense arm training with comparable effects. Therefore, robots are a significant aspect of the current therapeutic paradigm for improving the overall quality of neurologic rehabilitation [5].
For more information about neurorehabilitation, visit:
- Efficacy of technology-assisted gait rehabilitation in Parkinson’s disease
- Efficacy of placebo in managing pain for neurological disorders
- Neurorehabilitation in dystonia – a holistic approach
We kindly invite you to browse our Interview category: https://efnr.org/category/interviews/. You will find informative discussions with renowned specialists in the field of neurorehabilitation.
References
- Kuo CY, Liu CW, Lai CH, Kang JH, et al. Prediction of robotic neurorehabilitation functional ambulatory outcome in patients with neurological disorders. Journal of NeuroEngineering and Rehabilitation. 2021. doi:10.1186/s12984-021-00965-6
- Iandolo R, Marini F, Semprini M, Laffranchi M, et al. Perspectives and Challenges in Robotic Neurorehabilitation. Applied Sciences 2019. doi:10.3390/app9153183
- Chien WT, Chong Y, Tse MK, Chien CW, Cheng HC. Robot-assisted therapy for upper-limb rehabilitation in subacute stroke patients: A systematic review and meta-analysis. Brain and Behavior 10, 2020. doi:10.1002/brb3.1742
- Huang VS, Krakauer JW. Robotic neurorehabilitation: a computational motor learning perspective. Journal of NeuroEngineering and Rehabilitation 6, 2009. doi:10.1186/1743-0003-6-5
- Jakob I, Kollreider A, Germanotta M, Benetti F, et al. Robotic and Sensor Technology for Upper Limb Rehabilitation. Innovations Influencing Physical Medicine and Rehabilitation, 2018. doi:10.1016/j.pmrj.2018.07.011
- Rehmat N, Zuo J, Meng W, Liu Q, et al. Upper limb rehabilitation using robotic exoskeleton systems: A systematic review. International Journal of Intelligent Robotics and Applications 2, 2018, 283–295. doi:10.1007/s41315-018-0064-8
- Dehem S, Gilliaux M, Stoquart G, Detrembleur C, et al. Effectiveness of upper-limb robotic-assisted therapy in the early rehabilitation phase after stroke: A single-blind, randomised, controlled trial. Annals of Physical and Rehabilitation Medicine 62, 2019, 313-320. doi:10.1016/j.rehab.2019.04.002
One thought on “Robotic neurorehabilitation for the upper limb – new insights”