Improving control of dynamic devices relying on endogenous EEG-Based BCIS
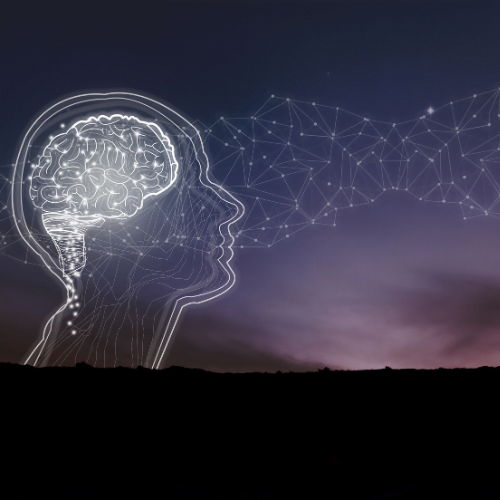
Author: Livia Popa
Keywords: Brain-Computer Interface, EEG, Motor Imagery, dynamic devices, endogenous, Brain-Machine Interface, control
Introduction | BCIs based on EEG
Controlling external devices with brain-computer interfaces (BCIs) based on Electroencephalogram (EEG) is a new approach in motor recovery technology. Nowadays, intuitive control of external devices is possible; endogenous paradigms are now based on commands generated by users autonomously, without other external stimuli, to enable exoskeletons, robotic arms, mobile robots, and wheelchairs. Given the complexity of endogenous BCI control over the dynamic devices and the many challenges faced in this field, the literature on the subject is vast, and a review that focuses on specific issues related to this kind of framework was needed. Recently, a review spanning publications through 2021 was published [1].
Literature Selection
The authors [1] focused on information regarding the control of dynamic devices based on endogenous BCI and, to ensure a high level of objectivity, a specific methodology was applied for selecting the papers on which their review is based; this methodology is PRISMA (Preferred Reporting Items for Systematic Reviews and Meta-Analyses), and it involved the following steps of selection:
- Defining eligibility criteria: only studies published as journal papers through 31st of December 2021 and not retracted were considered relevant due to the high level of research quality required for publishing; only studies on human testing and fully noninvasive scalp EEG were included and only papers presenting novel BCI implementation (not just potentially applicable SW/HW technologies, editorials or tutorials) were taken in account. Also, papers had to focus exclusively on endogenous BCIs (totally leaving out the exogenous signals), had to involve an active BCI (and not a passive one) that controlled an external, dynamic device, and not be dependent on any motor execution associated to the upper and lower limb, to be included in the review.
- Identifying the right sources for information and defining an efficient search strategy: the literature review was built with resources from three main databases: PubMed, Scopus, and IEEE Xplore, with some on-top papers recommended by experienced researchers in the BCIs domain.
- The selection process consisted of downloading and duplicate proofing, resulting in 2557 papers, as well as evaluating the abstract of each paper as a first filter, which then resulted in the exclusion of 2047, and only after reading the entire text from the remaining 510, for further refining of the data pool, which meant excluding the majority of the articles and having a final database amounting to 66 papers for the review, six of them being eligible from the total number of papers recommended by peers [1].
Figure 1 shows that half of the papers on which the review is based were published in the last three years, so the information is relevant for the contemporary context of BCI technology used for dynamic devices [1].

Device Control Types
There are two main types of device control:
- Synchronous control involves an established interval in which the user of a device can provide a command, and only at the end of that interval is the data assessed and the command implemented;
- Asynchronous control allows the user to issue commands anytime, and those commands are executed through data assessment performed at regular, very short intervals (fractions of seconds).
Most of the papers in the review [1] are focused on asynchronous control patterns. While synchronous control showed high accuracy, it also resulted in fragmented movements in dynamic devices when smooth movement is the ultimate goal in controlling exoskeletons, prosthetics, wheelchairs, and other such devices [1].
BCI Applications and Developed Paradigms
There were several types of devices controlled through BMI studied in the papers, and the frequency for each of them can be observed in Figure 2, mobile robots and assistive limb-movement devices (exoskeletons, orthotics, and haptic robots) were the most frequent, while unmanned aerial vehicles (UAVs) were among the least recurrent, together with prosthetics and pedaling machines.

There is a profound and significant correlation between the application a BCI is designed for and the endogenous model that is used, and the reviewed papers included the following models:
- Motor Imagery (MI) paradigms: the most common model present in the relevant literature, as it allows intuitive control of devices through the user imagining different actions, like walking, sitting, pedaling, flexion, or extending the hand. Patterns based on MI were observed to use up to four commands; they required serious training on the user for efficient use and were affected by a high rate of BCI illiteracy [2].
- Spelling and induced emotions: the spelling concept was the least common one, used only for one device in the whole database of papers and as a synchronous system, which proved itself to be an impractical approach because it needed the user to continuously spell a command for it to be implemented; self-inducing emotions is also impractical because it requires more psychological and mental effort than other frameworks and because of the impossibility to relate in a logical way emotions to navigational commands.
- Facial-movement paradigms have been proven to have the lowest BCI illiteracy rates, so they were suggested to be a feasible alternative to the other endogenous models. Facial movements (blinks, mouth, and eyebrow movements) send clear signal characteristics on EEG data, easily and accurately detectable.
- Multi-paradigm systems implied integrating MI with other models – merging attention detection, and MI classification resulted in a mixed framework used to control an exoskeleton and merging MI with facial expression to control a wheelchair.
Shared control is a solution developed for performing sudden actions on dynamic devices, like emergency stopping and obstacle avoidance, but also for semi-autonomous navigation. This solution consists of joining the output from a BCI decoder with the data transmitted by the environmental sensors, and it was presented as a viable system for controlling dynamic devices in almost 30% of the reviewed papers.
Since it is based on two merging dimensions – the user’s autonomy in driving the system via the BCI decoder and the actions following the feedback received from the sensors – shared control levels vary [3]. Figure 3, presented below, summarizes the shared-control approaches conferred in the analyzed literature:
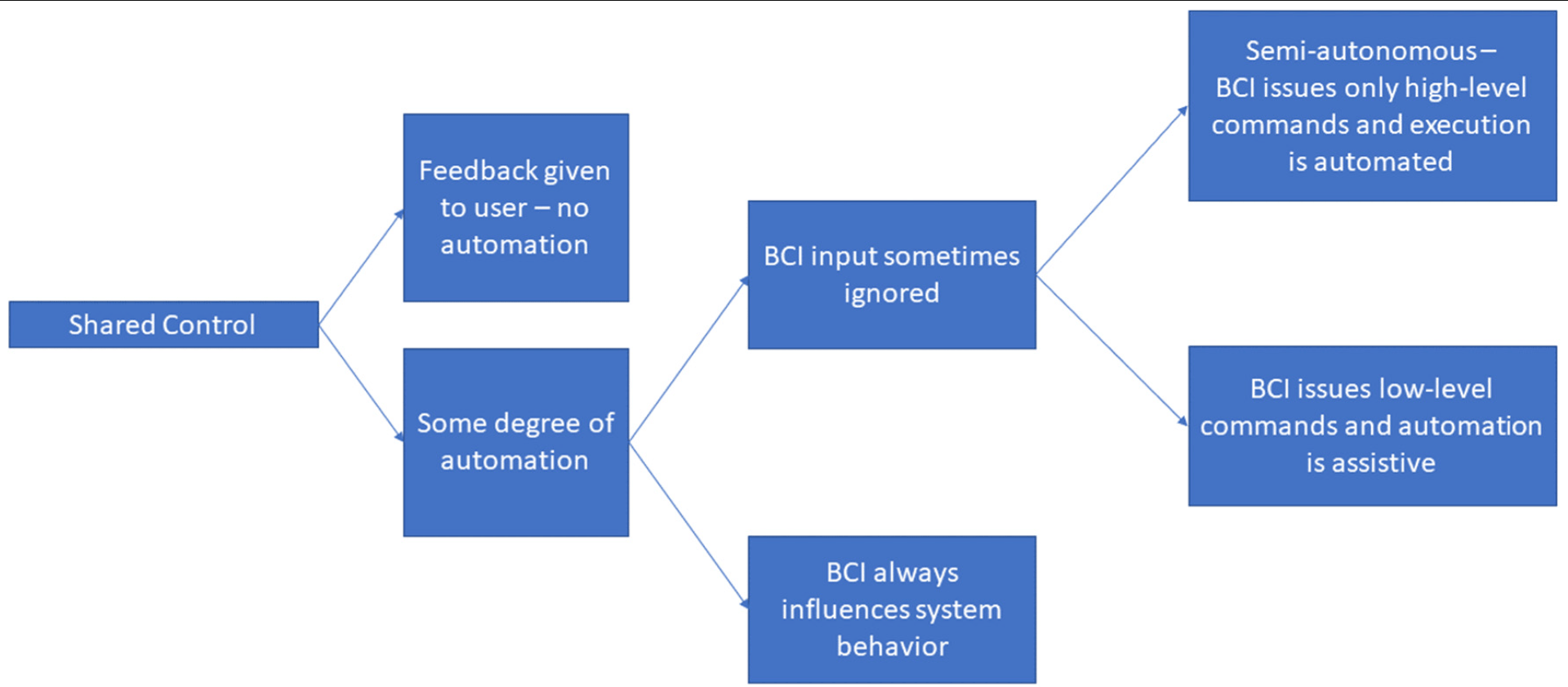
It is important to mention that while a higher level of shared control means a lower mental effort for the user, the possibility of the user’s will being ignored and surpassed by a semi-autonomous system has to be avoided and eliminated.
Increasing Control Stability in BCI Decoders
Most of the selected papers concentrated on control generated through signals from a BCI classifier, but because it has been observed that this kind of output is not sufficiently stable for driving external devices, some solutions were proposed for gaining more reliable control signals [1]:
- False-alarm approach: is based on the idea that changing one’s mental state in an instant is not possible, so the system is programmed to classify a change in the mental state detected in the classifier output as a false alarm at first and then validate it only if it is maintained for several successive classifier outputs.
- Smoothing approaches: are based on setting an average for the label probabilities that the classifier generates as an output in a determined range of time steps (2 to 8 steps in the majority of papers) to smooth the control signal.
In Pursuit of Higher Degrees of Freedom in Endogenous BCIs
The biggest challenge in working with endogenous BCIs is represented by the limited number of classes that can be defined with high accuracy. It has been shown that endogenous systems relying on mental commands were limited to four or even fewer commands, while a wide range of freedom degrees defines dynamic devices, so there have been several creative solutions for making possible the control of those devices by using endogenous BCIs:
- Sequential command paradigms consist of training a BCI classifier in a series of singular endogenous activities and commands generated through two such activities, performed in separate sequences (like first imagining the left-hand movement and after imagining the right-hand movement). This input is decoded, and the command to the device is issued.
- The finite-state machine is a concept that increased the number of commands even more, as it added a new coordinate to the previous model of sequential commands: finite-state machine, which links the commands to the current system’s state – a sequential command could start an idle wheelchair, while the same sequential command on a moving wheelchair could accelerate it.
- Hybrid BCIs (hBCI) is a framework that uses additional biosignals besides EEG for increasing the degrees of freedom; the reviewed literature presented this framework applied on quadcopters and their control and navigation included the addition of electrooculogram (EOG) signals for higher diversity in commands performed on the device [4].
- Menu navigation with limited commands is a concept that allows the device users to choose the actions of a dynamic device from a graphic menu that can be navigated with a limited number of commands, offering the user different control levels.
Error Handling for Obtaining Performance Corrections
As the BCI decoders, even the most advanced ones, can face misclassifications, or even mental state changes of the users (they cannot concentrate at times, for example), which, of course, affect their performance, generating errors in the handling of the device, confronting this challenge has become a topic of great interest.
A solution identified for this issue is associating error-related potentials (ErrPs) to BCI control, pairing MI and ErrPs to control dynamic devices. In the studies described, when the user of a device became aware of errors in robots/devices’ behavior, ErrPs spikes were present in the EEG signals, then decoded with a specific device, which caused the robot/device to correct its performance [5].
New Signal Processing Dimensions and Classification Methods for Performance Improvement
State-of-the-art BCI performance relies on the accuracy of EEG classification methods for good results in practical applications and for safe and precise control of dynamic devices that usually are meant to navigate through complex itineraries and conditions or to accomplish sensitive motor tasks. The focus in many of the papers was represented by new classification techniques for classification performance improvement, such as:
- Traditional machine learning features were used in studies for a better classification of the data collected from EEG signals; adding the common spatial paradigm (CSP), time-domain, time-frequency domain, and frequency-domain dimensions to the interpretation of MI EEG data results in increased accuracy of the classified data through the spatiotemporal characteristic these dimensions identified.
- Techniques based on deep learning rely on the principle of multi-layer processing of EEG data within deep neural networks (like spiking neural network (SNN) and convolutional neural network (CNN), that simulate the brain activity) for extracting crucial features that articulate specific commands on the dynamic devices [6].
- Integration of both traditional machine learning and deep learning methods that put together the outputs from machine learning and deep learning techniques in a complementary process that combined their classification results for a better accuracy level than the one obtained using only one of the two, thus generating an overall increased performance in the dynamic devices.
Conclusions
The central challenge in the dynamic devices’ domain is represented by the lack of practical command, which often requires facial expressions or spelling paradigms to control a system, but while these models are highly detectable, they can lead to counter-intuitive commands.
Most dynamic devices rely on MI frameworks. However, the MI systems showed a relevant BCI illiteracy degree and limited degrees of freedom, so a hybrid approach to the BCIs has been more and more present in the specialized literature and studies over the years, suggesting solutions like sequential commands and finite-state machines for an improvement in control potential [4].
Shared control is a valuable addition to controlling systems for efficient navigation in complex environments because it gives the users the possibility for emergency stopping and obstacle avoidance with a lower mental effort. Error-Related Potentials signaling is another solution identified for confronting eventual errors in BCIs and correcting them for more stable control and smoother handling of endogenous BCI-based dynamic devices [3].
Further research on all these topics and endogenous EEG-based BCIs for dynamic device control is an ever-ongoing process, with studies directed towards including more and more subjects recruited from the directly impacted population. Gathering relevant information about the efficiency and relevance of studies also requires including surveys focused on user experience for improvements and optimal follow-ups [1].
For more information on neurorehabilitation, visit:
- What is the impact of early life on brain and cognitive reserve?
- Does exercise induce neuroplasticity?
- Efficacy of placebo in managing pain for neurological disorders
We kindly invite you to browse our Interview category: https://efnr.org/category/interviews/. You will find informative discussions with renowned specialists in the field of neurorehabilitation.
References
- Padfield N, Camilleri K; Camilleri T, Fabri S, Bugeja M. A comprehensive review of endogenous EEG-Based BCIs for dynamic device control. Sensors 2022, 22, 5802. https://doi.org/10.3390/s22155802.
- Abu-Rmileh A, Zakkay E, Shmuelof L, Shriki O. Co-adaptive training improves efficacy of a multi-day EEG-based motor imagery BCI training. Front Hum Neurosci 2019; 13:362. doi: 10.3389/fnhum.2019.00362.
- Cao L, Li G, Xu Y, Zhang H, Shu X, Zhang D. A brain-actuated robotic arm system using non-invasive hybrid brain-computer interface and shared control strategy. J Neural Eng 2021;18(4). DOI: 10.1088/1741-2552/abf8cb.
- Saha S, Mamun KA, Ahmed K, Mostafa R, Naik GR, Darvishi S, Khandoker AH, Baumert M. Progress in Brain Computer Interface: challenges and opportunities. Front Syst Neurosci 2021; 15:578875. doi: 10.3389/fnsys.2021.578875.
- Yousefi R, Rezazadeh Sereshkeh A, Chau T. Development of a robust asynchronous brain-switch using ErrP-based error correction. J Neural Eng 2019; 16(6):066042. doi: 10.1088/1741-2552/ab4943.
- Tayeb Z, Fedjaev J, Ghaboosi N, Richter C, Everding L, Qu X, Wu Y, Cheng G, Conradt J. Validating deep neural networks for online decoding of motor imagery movements from EEG signals. Sensors (Basel) 2019; 19(1):210. DOI: 10.3390/s19010210.